3 Our Predictive Brains
Outline
The Predictive Processing Framework
Following up on the previous two computationally-oriented chapters, we now come to the pièce de résistance: a computational theory of a canonical cortical computation that has been hailed as providing a unified brain theory. This theory goes by a number of names, such as Predictive Processing, Predictive Coding, and the Free-Energy Principle. The basic idea of the theory is that the cortex of the brain is composed of hierarchies of neural networks that are principally oriented toward predicting what’s going to happen next. The structure and connectivity of these neural networks are so regular that they have been called canonical microcircuits. Because these microcircuits are so widespread and systematically interconnected through the cortex of the brain, it has been said that “anticipation is at the core of cognition”.
Below is a schematic diagram of what this circuitry might look like.[1] First, note that information flows in two directions, as denoted by the blue and red lines. The blue lines, and the corresponding blue-shaded components of the diagram from which they emanate, carry “top-down” information. This information represents what you already know about the world. Or, in other words, it contains your internal models of the world. The red lines, and the corresponding red-shaded components of the diagram from which they emanate, carry “bottom-up” information. This is information that originates in your senses. However, what’s passed “up” the cortical hierarchy (e.g., from lower-level areas like V1, the primary visual cortex which specializes in line detection, to secondary visual areas like V4 which specializes in color processing) isn’t the sensory information itself, but how the information deviates from, or was unpredicted by, a local cortical model of the world. This process of model matching occurs at multiple levels in the cortex of your brain until your brain is either satisfied that it has now correctly predicted incoming sensory information, or revises its models so that subsequent predictions will be more accurate.
Let’s unpack what’s happening here through a simple example. Suppose I told you there was a house located off to your right-hand side. Because you have a lot of experience looking at houses, your brain already has very refined brain models of what a house looks like. You will (usually implicitly, unconsciously) expect to see certain visual features when you look to your right. For example, you will expect to see a structure arising vertically from the ground to an approximate height (higher than a fence but lower than an office building, on average). That structure will have an array of structures built into it, such as windows and a door. These structures, in turn, will be composed principally, if not entirely, of vertical and horizontal lines, marking the top, bottom and sides of the windows and doors. When I tell you to expect a house to your right, high-level areas of your brain which process house-information (in other words, which contain housing models) will become activated. For example, the parahippocampal place area (PPA) codes for spaces such as houses. The active house models of your PPA will then prime lower-level areas, via top-down connections, to expect house-related features. Since windows and doors are composed of corners, relevant corner-related neurons in V2 will become primed. And V1 will expect to “see” vertical and horizontal lines, rather than an assortment of diagonally-oriented lines.
To the extent you *perfectly* predicted the house you expected to see, there would be no error in your model predictions to pass up, and what remains in the brain are the effectively-confirmed representations[2] of the house already generated by your brain. In other words, on this account, sensory input simply confirms your expectations and you see what you expected to see.
Of course, it would be nearly impossible to *perfectly* predict the house you expected to see. Even if you knew the house was a very familiar house (like your current house or your childhood home), viewing it from different angles in different lighting conditions with different contextual features (like overgrown shrubbery in front) will create slight differences compared to what you’d expect. These “prediction errors” will pass up to higher levels of your cortical hierarchy so that your brain can generate a more fitting model of the current input.
In this sense, prediction error serves a similar role to what we may have thought, a priori, the sensory input itself would do: enable the creation of an appropriate representation of the world. Indeed, if we had no prediction at all about what we were about to see, the difference between (a null) model and (the sensory) data would just be the data itself. In other words, with no prediction model, the sensory data and the “error signal” are one and the same.
Most commonly, we neither have completely perfect nor wholly absent predictions about the world around us. There will always be some iterative dynamical processing over time between layers of the cortex to generate and test predictions about sensory input. But in all cases—according to this theory—input principally confirms or disconfirms your models (or, more accurately, the disconfirmation of models drives the selection of alternative models until a good-fitting model is confirmed). You are never building a representation of a house (or anything) from the “ground up”, as though you had never seen a house before, except in those cases where you’ve literally never seen such a thing before (e.g., in infancy or with very foreign objects).
Note that this is very different from where we left off in the last chapter, showing how a hierarchy of “and networks” could come to create a representation of an object (in that case, an “E”, and here, a house) from the bottom-up, using “feedforward” neural networks. This is true. But it’s not how your brain is thought to generally work. Now we’re examining top-down activation, via “feedback” neural networks. Top-down connections change everything. To the extent that you have already encoded a representation of an object in the internal models of your brain, and the object of that representation is now encountered in the world, there’s no need to try to reconstruct that representation. It would be much more efficient to simply reactivate and confirm your already encoded knowledge. It would also be more energy-saving to expand your existing models with the new versions of objects (like different houses) that you are encountering.
As another example, let’s imagine what happens if you looked for the house I told you to expect to your right-hand side and saw instead something completely unexpected, like a futuro house!

Your first response might be close to a startle response: “What the heck is that?!” You would slow down, look again, perhaps even stare (just like babies who are surprised). That’s a house? How? Why? Who? You have comparatively little information to bring to bear on this strange sight. You’d have to piece together what’s going on to understand this strange configuration. In neural terms, your brain would be generating error signals at most levels of its cortical hierarchy for representing houses. These error signals would then be used to revise your internal house-model: sometimes, houses can look like UFOs! And so, the next time you encounter such a house, you’ll be less surprised.
Note what happened in this example:
- The unexpected grabbed your attention, almost certainly interrupting any other activities you may have been engaged in at the time.
- You took time to try to figure out what exactly you were looking at.[3]
Another way of saying this is that prediction errors can trigger an alerting and orienting response. Often, a key way we notice the predictions our brain is making is when things don’t exactly happen as predicted. Here’s an example I’m quite fond of, courtesy of Jeff Hawkins:
Demonstration 3.1
Read the following quickly:
What did you notice? Did your mind automatically fill in the word “sentence”. Why? As you were reading, your brain was developing and refining a model of the meaning of this sentence, based on the unfolding grammar and word semantics, to predict its likely ending. Because that prediction is encoded in a model which sends input to lower levels of the cortical hierarchies within which it is embedded, you would be primed to hear the word your brain predicted. Indeed, in the absence of any word, you noticed that this gap was filled by your brain’s prediction: the word just came to mind! Of course, then you slowed down (and hopefully found the example amusing!) when you realized the word wasn’t actually there but indeed fit perfectly. Your brain’s error signals (driven by the discrepancy between expecting the word “sentence” and there in fact being no ending to the sentence) grabbed your attention. But critically, that prediction would occur even if the word “sentence” actually occurred. You just wouldn’t be aware of it because your brain’s prediction would be quickly confirmed by the visual input (and “auditory” input if you read to yourself in your head), and so you’d readily move on to the next sentence to read. We don’t notice how ubiquitous the predictions are that our brains are generating because they are usually either correct or good enough. Only when they are insufficient, incorrect, or in some way surprising are additional attentional and information-processing resources recruited.
In the absence of the word “sentence”, it was almost as if your brain hallucinated the word “sentence”. Indeed, we often “see” things that aren’t there, even when we know they aren’t really there. Perhaps the most famous examples are seeing a “man in the moon” or various shapes in clouds. This propensity for bringing our existing knowledge of the world to bear on otherwise meaningless input has been called pareidolia and patternicity. My favorite example is of a grilled cheese sandwich which sold for $28000 because it appeared to have the face of the Virgin Mary on it!
Note that if the word “sentence” had appeared more forcefully in your mind’s ear, you might indeed perceive it as a hallucination. Certain conditions (like schizophrenia) and certain drugs (like psychedelics) increase the likelihood of perceiving a hallucination. Hallucinations are a kind of uncontrolled perception.
But even more radically, the fact that sensory input is merely used to confirm perceptual representations already generated by your brain, rather than create them, means that we can think of perception as a kind of controlled hallucination! Reality is not what it appears to be. Reality is what appearances confirm we already see it as.
For more on this fascinating idea of perception as a controlled hallucination, see this TED talk by Anil Seth:
To summarize, the architecture of our brain’s cortex is such that we are constantly making predictions about the world around us. So far, I have discussed this principally with respect to visual processing, which is also the domain on which most neuroscientific research has focused. But predictive processing reaches beyond vision. Literally. It applies to movement as well. For example, we’re constantly making predictions about the sensory and motor consequences of placing our feet in particular positions on the grounds as we walk. We only notice these predictions when they go awry: a lip on the sidewalk catches the toes of one of our feet and we trip (usually looking back to figure out What…The…Heck…Happened!?!). We’re constantly making interpersonal predictions about what people will say or do next. This is particularly noticeable if someone doesn’t say or do what you thought they would. In short, our interaction with the world (be it physical, social, cultural, linguistic, or otherwise) is mediated by and in reference to our predictions, which reflect our learned models of those worlds.
Activity 3.1: Prediction Diary
As discussed above, we are always making predictions about the world around us. Sometimes we catch ourselves having made a prediction—often when a prediction wasn’t right. For the next week, keep a running list of predictions that you notice your brain making. Jot down a short phrase to help you remember the prediction. Just like with trying to remember dreams, the act of trying to notice your brain’s predictions will make you more attuned to them.
As you notice your brain’s predictions, think about how you noticed that your brain had made the prediction. Were you aware of having an expectation before an event occurred? Did you only notice the prediction when something unusual or surprising occurred?
Day | Predictions noticed: | How you noticed: |
Day 1 | ||
Day 2 | ||
Day 3 | ||
Day 4 | ||
Day 5 | ||
Day 6 | ||
Day 7 |
Advantages, Consequences, and chatGPT
The ultimate reason our brain works as a predictive processor is because it has served us well over evolutionary time. One advantage of predictive processing is that it allows us to compensate for otherwise “noisy” information. Noisy means that information is somehow incomplete, ambiguous, or distorted. For example, if you look out the nearest window, you’re likely to only see parts of objects: parts of trees, people, buildings. But you’re not confused by floating trees, legless people, or oddly shaped building cutouts. You immediately and unconsciously understand that those partial data sets correspond to models of whole trees, people, and buildings. Most of the information in our environment is noisy in just this way. For another example, we can usually hear what other people are saying despite all kinds of other auditory noise in the environment altering the patterns of sound waves actually hitting our eardrums. But we are generally unaware of how noisy our information environments are precisely because we are evaluating model predictions rather than constructing sensory-based representations. In other words, if the data about a tree is sufficient to confirm “tree”, seeing part of a tree will not elicit an “alert and orient” response.
One of the most famous examples of how “top-down” knowledge enables perception can be seen here. If you’ve never seen this before, you have no idea what you’re looking at! But at some point, you see it. Or you read what is there (scroll further down the page at the last link). And now, you can’t NOT see the object. You’ll never be able to go back to not knowing what is depicted in this particular image. You’ll never go back to having to piece together what’s going on. You will automatically recognize what is being depicted.
In the absence of an internal model of what you are looking at, you remain in “alert and orient” mode, piecing together what is going on. This takes a lot of time, as you may have experienced in the Dalmation example. Predictive processing allows you to 1) compensate for noisy environments and 2) very quickly recognize what you are experiencing. You can easily imagine how this might be advantageous in a world of threats, like predators that might be hungry!
A third advantage of predictive processing is that predictions can be wrong. Why might this be an advantage? By making a prediction, and then confronting a different reality, the discrepancy between your prediction and that reality can be used as a training signal to improve your models. Over time, your models, and therefore your predictions, will get better and better. But they also always contain within them the seeds for possible learning and improvement.
Think Critically 3.1
Our brains are not the only intelligent devices that operate by making predictions. In November 2022, ChatGPT was unleashed on the world. Like the brain, chatGPT works by making predictions. More specifically, ChatGPT types out its best predictions about how to respond to user-entered prompts or questions as well as how best to structure its response. The screenshot below of my interaction with chaptGPT likely mirrors your own experience with the demonstration earlier in the chapter!
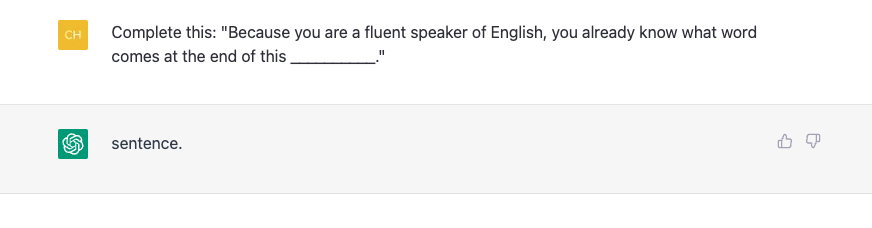
The architecture of chatGPT shares with the brain its use of deep (many-layered) neural networks, trained using multiple types of learning algorithms (e.g., reward learning and supervised learning, to name two), to modify a very large number of parameters. While chatGPT’s detailed architecture is otherwise different, we know from the first chapter that any entity with a large number of components and interactions between them are candidate structures for producing emergent behavior. ChatGPT currently has 175 billion parameters. In comparison, our brains have at least 100 trillion parameters (if we only count synaptic connection strength as a parameter). This number of parameters may well be reached by the next generation of GPT! It will be interesting to see what emerges then!
Activity 3.2: ChatGPT
Given some of the similarities between our brains and chatGPT, an instructive way to appreciate the powers and perils of prediction-based computing is to critically examine chatGPT.
- What are the strengths of chatGPT?
- What are the weaknesses of chatGPT?
- How do the strengths and weaknesses of chatGPT correspond with our own?
Begin by brainstorming these questions, individually or with others. Then, enter these questions into GPT to see how it responds! You can also enter the disadvantages you came up with in the last exercise to see to what extent chatGPT may also suffer from them. Finally, come up with and enter other questions querying the relationship between chatGPT and the brain.
Prediction and Curiosity
Much is being written about how chatGPT (and its future incarnations) has and will change the nature of education. What’s also worth considering is how education, regardless of its form, interacts with the architecture of our brains as prediction processors. I’ve written an article with colleagues about how education can shape the conditions for lifelong learning and critical thinking. How? When you learn, whether it’s new facts or new skills, that learning is instantiated in your brain. Your learning-altered neural networks will encode new and revised models about how the world works and how to interact with it—most directly in the area of study in which you may have specialized. Your newly revised mental models will, in turn, alter the range of predictions you make about the world as you interact with it. When you encounter a claim—from a friend or colleague, or on social media, for examples—that claim may strike you as somehow “off”. It doesn’t sound quite right to you. That not-feeling-right comes from the errors generated by a discrepancy between this claim and your internal models. The next step, according to the Prediction Appraisal Curiosity Exploration (PACE) framework is to appraise whether this prediction error is something that you’re curious to get to the bottom of or not. If so, that curiosity will then motivate you to figure out why a claim doesn’t sound right, which could involve either understanding and then explaining the error of the claim, or revising your own understanding to incorporate your new understanding of what is true. In short, education shapes your internal models, which alters the kinds of predictions you subsequently make and the kind of prediction errors you later experience. This computationally- and neuroscientifically-grounded account helps explain why we might engage in critical thinking in our everyday lives, when we’re not required to do so by an instructor. As Jane Halonen, a critical thinking theorist, has written, “Surprise is at the basis of the disequilibrium that triggers the critical-thinking process; the thinker engages in critical thinking to reduce the feeling of being off balance or confused”. Surprise arises from an information gap, from a prediction error. But surprise itself isn’t enough. Critical thinking is much more effortful than simply relying on the cognitive heuristics that govern much of our thinking. We have to be motivated to do it. As the PACE framework articulates, curiosity—which follows an assessment of a prediction error—provides that motivation! We also tend to better remember information we learn when we’re in a state of curiosity. This means that curiosity not only motivates us to learn but helps encode that learning, further shaping our internal predictive models and the kinds of new encounters that might provoke a new bout of curiosity and learning.
While we may sometimes have conscious access to the nature of the predictions our brain is making, particularly when those predictions are wrong, curiosity is by definition a subjective state. Curiosity may provide a privileged window for exploring our internal mental landscape—or, more accurately, the interaction of our internal models with our environments.
Activity 3.3: Curiosity Journal
Curiosity connects our past and our future. It can arise in response to the gaps between what we now know and what we don’t yet know. When we’re curious, we feel motivated to explore, to close these gaps, to expand our models of the world. By tracing and pulling on the threads of curiosity, we can learn more about ourselves and grow into our future. I’m reminded of the comparative mythologist Joseph Campbell who distilled the core lesson of countless myths from peoples across the world as: “Follow your bliss”.
Let’s get curious about curiosity by keeping a curiosity journal for a period of time. Note the things you are curious about over the course of a week or a month. Do the things you are curious about share similarities? Can they be categorized in some way? What do they indicate about the kinds of information you’d like to have? If you’re a student still searching for a career path, in what directions might your curiosity be pointing you? Perhaps, as you are considering future life paths, curiosity can be your muse.
We are having experiences all the time which may on occasion render some sense of this, a little intuition of where your bliss is. Grab it. No one can tell you what it is going to be. You have to learn to recognize your own depth
― Joseph Campbell, The Power of Myth
- A number of scholars have theorised that prediction is “at the core of cognition” and a “canonical cortical computation” that may provide a “unified brain theory”.
- The essence of the predictive processing framework is that our brains have developed a range of internal models which generate predictions about incoming information. Prediction errors are passed up the cortical hierarchy to aid the selection or formation of better fitting models.
- Prediction errors can trigger an “alert and orient” response, which is one way to tune into the predictions your brain is constantly making.
- Predictive processing helps make sense of noisy informational environments, speed up recognition of environmental stimuli, and provide error signals that drive learning.
- As a consequence of predictive processing, we may perceive patterns and objects that are not really in the environment. We may also see interpersonal exchanges through the lens of our expectations, biases, or stereotypes.
- ChatGPT is another prediction engine. Interrogating the similarities and differences between chatGPT and our brains may be an instructive way to better learn about the strengths and limitations of prediction.
- Predictive processing provides a framework which may explain how education can facilitate critical thinking and lifelong learning.
- Prediction errors can spark curiosity which drives exploration and learning.
Media Attributions
- PredictiveCodingX2 © YON - Jan C. Hardenbergh is licensed under a CC BY-SA (Attribution ShareAlike) license
- Futuro_Pinakothek_München_6493 © Henning Schlottmann (User:H-stt) is licensed under a CC BY-SA (Attribution ShareAlike) license
- ChatGPT Screenshot © Christopher May is licensed under a CC0 (Creative Commons Zero) license
- Additional circuit diagrams can be found in the papers linked to in the opening paragraph. The precise ways in which predictive processing operates in the brain is still a matter of very active investigation. ↵
- For the philosophically minded or versed reader: whether the brain traffics in “representations” is a matter for debate. But it doesn’t substantively alter the story here. ↵
- Note that the unexpected doesn’t ALWAYS grab our attention. This will be discussed at the end of the next chapter. ↵
- Just about every feature of our brain’s architecture is such that it will offer advantages for certain kinds of tasks or in certain kinds of environments, and entail disadvantages for other kinds of tasks or environments. For a greater discussion of the tradeoffs inherent in our brains (and how these help make us unique!), I highly recommend a book by my friend Dr. Chantal Prat, The Neuroscience of You. ↵