7 On Methods and Models
Outline
See: Key Takeaways
Methods
Demonstration 7.1
Take a look at this image. If someone pointed at it and asked you, “What is this?”, what would you say?
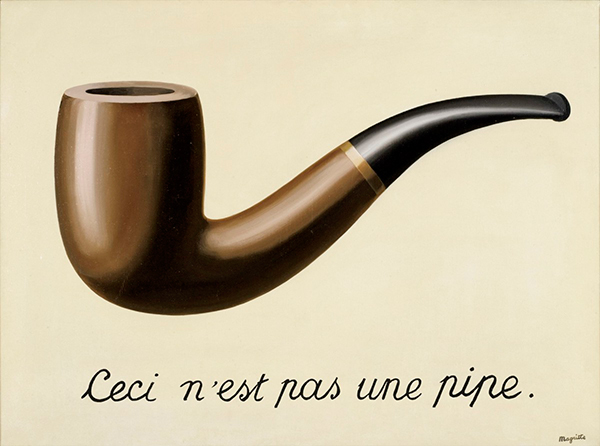
Your immediate response is likely to be “It’s a pipe”. (Accompanied by a curious look at such a strange question.)
You might be unsure how to answer, though, if you can read French. The caption says, “This is not a pipe”.
Rene Magritte’s famous painting points to the differences between the representations of objects and objects themselves. Magritte explains: “The famous pipe. How people reproached me for it! And yet, could you stuff my pipe? No, it’s just a representation, is it not? So if I had written on my picture “This is a pipe”, I’d have been lying!”[1]
Magritte’s representation of a pipe approximates one dimension of a pipe—namely, its visual appearance—but differs in other dimensions, such as its affordances. This is captured in Magritte’s retort that you could not use the representation of a pipe to smoke, nor would you even try, since we recognize paintings as having different possibilities of action. This is “the treachery of images”. Images only partially represent their object. We could say the same of words, which we would have used to describe the image as being of a pipe, rather than of a representation of a pipe.
Why does this matter?
We have a tendency to confuse the relationship between “maps” of a “territory” and the territory itself. We may conflate representations (the map) with the things represented (the territory), just as happened if you responded “It’s a pipe” when asked what you saw above. In that case, we can clearly see—when it’s pointed out—the difference between the object and the representation of the object. But understanding the relationship between the map and the territory is much more difficult with more sophisticated representations. Like brain images.
Press reports of studies producing images of brain activity, like the one below, are typically simplistic, sensationalized, and misleading. This is in no small part because brain images are the product of a complex series of processes and computations which would be very difficult (and off-putting) to accurately yet succinctly describe with each press report. However, when such overly simplistic reports are coupled with a widespread fascination with how the brain works, the result is the proliferation and persistence of neuromyths about how the brain works.
Consider the image below. A headline summarizing the research which produced this image might read: “Research shows the brain areas associated with memory”. Tacit in such a summary is how this image was produced: that researchers asked participants to engage in some kind of memory task, and the resulting brain image reflects the brain activity observed as participants were doing that task. But such an understanding would be very incorrect.
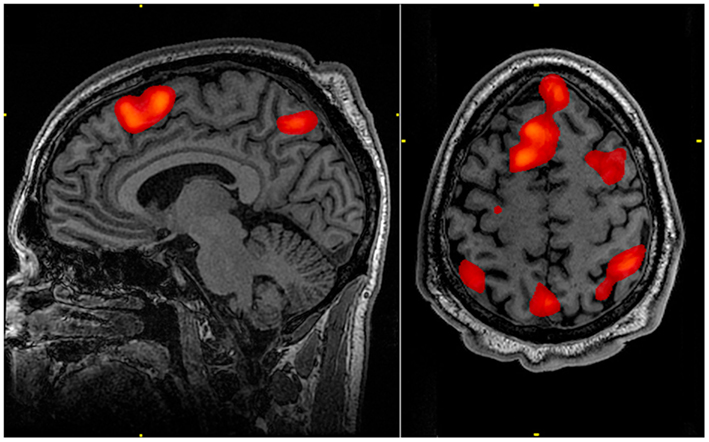
If that really was an approximately correct representation of the researchers’ experimental method, the resulting brain activity would be more likely to look like this image:
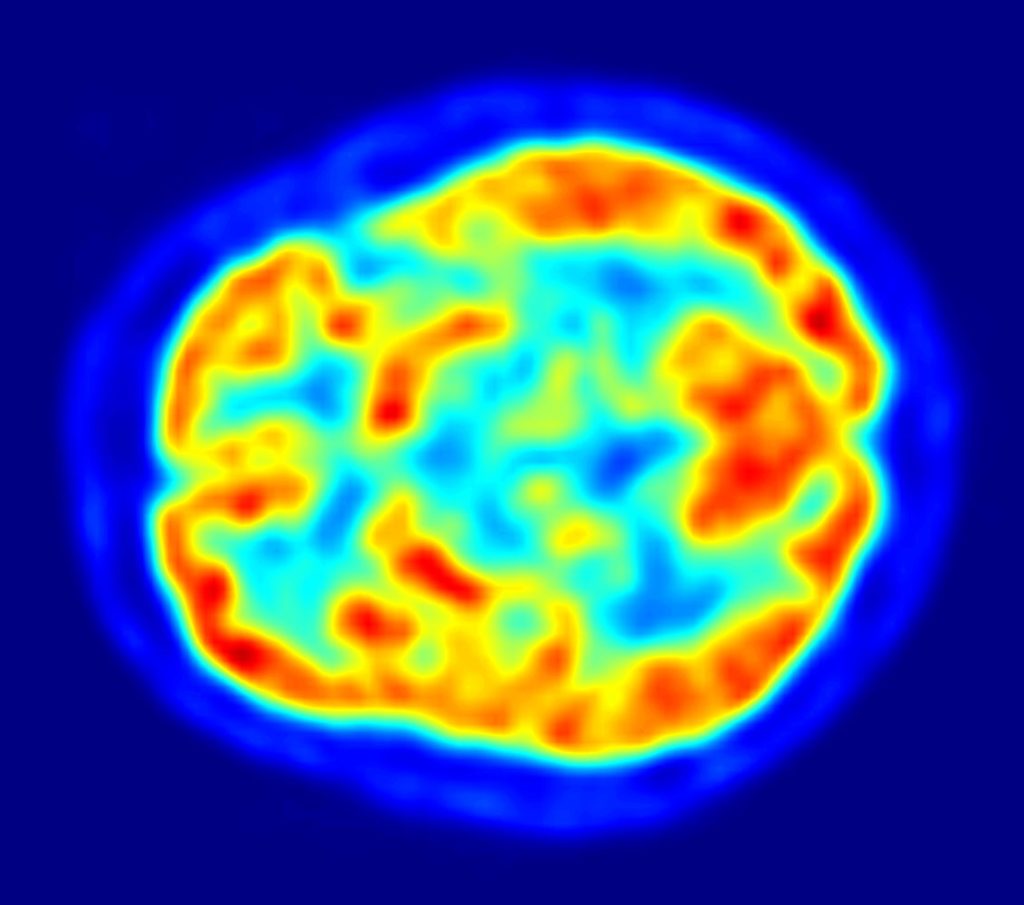
This image reflects the reality that at any given moment, most of our brain is active at varying levels.[2] While someone is engaged in a memory task, they are also engaged in other activities, such as processing sights, sounds, emotions, and other thoughts, while regulating their behavior—to name just a few activities with which they would be simultaneously preoccupied.
In order to isolate the areas of the brain associated with memory, whole-brain activity during a control task must be subtracted from whole-brain activity during a memory task. The idea behind this subtraction method in neuroimaging is that, over many trials, the average of “other” activity in one condition (viz., processing miscellaneous sights, sounds, emotions, and other thoughts) will be the same as the average of “other” activity in the comparison condition. Subtracting those from each other eliminates them from the resulting brain image. What remains in a brain image, then, should be the task differences between the experimental and control tasks. This is captured in the more accurately written caption of the image showing memory-related brain activity: “The highlighted regions showed significantly different activation between an individual performing a 1-Back [memory] task versus a 2-Back [memory] task”.
If you didn’t know that the subtraction method is a critical part of how neuroimages are produced,[3] you might well interpret images of brain activity as generally supporting the neuromyth that we only use 10% of our brain.
Activity 7.1
The previous section introduced the problem of overly simplistic representations of brain activity in the media. In this activity, you’ll see an example, which you are invited to explore afterwards.
Begin by watching this news piece: Is the secret to love in your brain? Then, turn to the questions below.
Brain images, such as the fMRI and PET images above, are far removed from being anything like the photograph of brain activity that they appear to be. Not only are brain images derived using the subtraction technique, but they represent an indirect and imprecise measure of brain activity: blood flow. The basic idea is that just as muscle tissue needs more blood during and after periods of use (e.g, to supply oxygen and glucose), so too does brain tissue need more blood during and after periods of use. fMRI and PET track blood flow in different ways, but both measure blood, rather than neural activity. Compared to the firing of neurons, which happens on a millisecond time scale, blood flow is very slow! It takes several seconds for blood to arrive in areas where neurons have just been active.
As a result, brain images have peculiar strengths and limitations as representations of brain activity. While they have relatively high spatial resolution, such that you can see which parts of the brain are more active during one task compared to another, they have relatively low temporal resolution, or detailed information about when those brain areas were active. Just as with the representation of a pipe in Demonstration 7.1, there are certain dimensions of the brain that neuroimaging techniques like fMRI and PET can capture well and certain dimensions that will be omitted or distorted.
This is true for all representations. If a representation captured all dimensions of the object(s) represented with perfect fidelity, it would instead be a recreation rather than a representation. Indeed, the point of a representation is generally to help make relevant dimensions of the thing represented more accessible. A map is a valuable structural representation of territory precisely because it has good spatial resolution and—unlike the territory itself—can fit in your pocket!
Because representations highlight only limited dimensions of an object, neuroscientists use multiple types of representations to get a more comprehensive understanding of how the brain works. For example, a complementary method to neuroimaging would be one which has high temporal resolution. This is provided by electroencephalography (EEG), which records the millisecond by millisecond activity of populations of neurons in the brain. The tradeoff for high temporal resolution in an EEG model, however, is relatively low spatial resolution. Researchers can get a precise timeline of when different information-processing operations happen in the brain, but have to estimate in a cruder way where those processes are occurring. By conducting research using both fMRI and EEG (which, for technical reasons, is usually done in separate studies rather than at the same time), a fuller structural and dynamic understanding of the information-processing landscape of the brain can be unearthed.
The figure below shows an array of different neuroscientific methods, each of which engenders a different tradeoff between spatial and temporal resolution. They also differ in portability. PET and fMRI have very low portability because the research must be done in a dedicated location containing the scanner and participants should remain still during the experiment. Other methods are either more easily carried out in diverse locations or allow a greater range of movement of the participant.
For brief introductions to additional methods used to study the central and peripheral nervous systems in humans, see here and here. Introductions to neuroscience also often highlight the complementarity of different methods (which can originate in different disciplines) used to understand nervous systems.

Models
In the previous section, we discussed representations and select methods for producing brain representations. In this section, we’ll broaden the discussion of representation to a discussion of models. While the term model can be used in multiple ways (e.g., a model airplane, a fashion model), models used in the sciences are often representations of systems that help describe, explain, and predict the functioning of that system. As representations, models are also partial approximations; partial approximations which foreground theoretically relevant dimensions to advance understanding of the modeled system. As the well-known aphorism goes:
Similar sentiments are articulated in the philosophy of science, where, for example, false models [are] means to truer theories. On this view, it is not a problem that models are wrong. Indeed all models that are not replicas will necessarily fail to instantiate or incorrectly instantiate some aspect of the modeled domain. For example, if you want to understand and predict population change dynamics, it’s useful to use models of differential equations. The equations capture dynamics that, in “the real world”, are instantiated by people, but the advantages afforded by a mathematical model are clear enough.
Much of this book—indeed, all of the book, as I’ll show—has been about models. These models complement the range of theoretical, experimental, and methodological models you would be more likely to be introduced to in a neuroscience or biopsychology course.
In Chapter 1, cellular automata models were used to demonstrate and explain emergence. We saw that these models could produce patterns similar to the striping pattern of zebra fur and the organization of ocular dominance columns in brains. Though the instantiation of cellular automate rules would be physiologically quite distinct in zebra fur and ocular dominance column organization, cellular automata models highlight fundamental processes that both may have in common. The chapter concluded by pointing out that brains (and perhaps even the entire universe) could be simulated using cellular automata. But cellular automata may not be the best-suited type of model for explaining and predicting information processing in the brain.
Chapter 2 introduced neural networks as models of information processing. An advantage of these models is that they help to bridge models of how neurons work with models of how brain regions work. It’s not at all obvious how neurons, which fire or not depending on things like ion concentrations and neurotransmitter bindings, could produce something like object recognition in visual cortices of the occipital and temporal lobes. Relatively simple neural network models are pitched at the right level of abstraction to provide an explanation. However, as artificial neural networks become larger and larger, they can be as difficult to understand as the brain itself. Indeed, deep learning in very large multilayered neural networks has been used to successfully perform object recognition (which our smartphones increasingly use to recognize our faces and categorize our images). But it’s often unclear how exactly machine learning has produced such artificial intelligence. These large-scale models, while useful in their own right, can be poor as scientific models if they don’t help explain or predict intelligent behavior.
Chapter 3 introduced a computational theory (model) of the brain according to which the cortex of the brain is fundamentally involved in making, checking, and correcting predictions. These predictions arise from internal models, just as good scientific representational models generate predictions about data that should be observed if the model is true! The chapter highlighted several advantages of a neural architecture that enables model-based predictions of sensory input, such as compensating for noisy environments and accelerating perceptual recognition. Like all models, our predictive models come with certain limitations. For example, we expect people to behave and situations to unfold as they have in the past and may therefore miss or minimize the extent to which the present in fact differs from the past. This is exacerbated in depression, where it can feel like our situation won’t improve. Alternatively, our models can reflect biases we grew up with, perhaps explicitly communicated by others or implicitly conveyed by a restricted range of experiences. Learned predictive models can only be as good as their “training data”. We are, arguably then, predisposed to these kinds of biases, just like machine-learning neural network models which also exhibit them.
Chapter 4 further explored “biases” in brain processing, this time resulting from difference detection and enhancement processes, as well as attention. “Biases” is now placed in quotation marks to highlight that biases are value-neutral. Biases can be negative, in the sense implied in the last paragraph. Or biases can be positive, such as enhancing differences in order to facilitate object recognition in the visual system. Biases—whether positive, negative, or neutral—follow from the inherent partiality of model representations. The chapter further presented selective attention as a “biasing field”. Attention spotlights what is being attended to and, as a consequence, inhibits awareness of other phenomena. As a result, there are dynamics to which we are either situationally or dispositionally blind.
Chapter 5 extended network models beyond neural networks. All levels of human organization, from microscopic intracellular processes to macroscopic sociological processes can be thought of and modeled in terms of networks. Many networks themselves share certain organizational properties, such as having a small-world structure. This means that network models at one level of organization could be used to generate hypotheses about analogous processes at another level of organization. There can be complementarities between, for example, social networks and brain networks. Like cellular automata models, network models can be applied to quite distinct phenomena, potentially highlighting otherwise invisible parallels between them. Of course, different levels of organization also have distinct ontologies with unique structures, properties, and relations. This is why there are different disciplines, each with their own models of understanding. All models, then, require care in determining what they can and cannot explain and predict.
Chapter 6 drew on Taoist and Buddhist models to explore health and well-being. The taijitu model of a harmonious and dynamic relationship between antagonistic forces served to highlight an analogous relationship between the sympathetic and parasympathetic nervous systems. A similar dynamic was also seen between the default-mode network and task-related networks. Buddhist models of suffering and its alleviation were connected to theoretical and empirical psychological models of the relationships between contemplative practices and eudaimonic well-being. Models, understood as such, facilitate analogical reasoning and, by extension, interdisciplinary exploration.
The models presented throughout this book can be classified into several types. For example, cellular automata models are computational models. These kinds of models typically consist of states (e.g., 0 and 1) and transition rules between states. The dynamic consequences of these states and rules are worked out using computer simulations. The integrate-and-fire model of a neuron is a mathematical model. These kinds of models can either be examined formally (e.g., developing mathematical theorems proving or disproving some claim) or can also be embedded within computer simulations, as is done in larger-scale neural network models. The predictive processing framework has been examined with multiple types of models: computational, mathematical, experimental, and verbal. Experiments are types of physical models because experiments involve the careful construction and control of testing stimuli and environments. Verbal models articulate relationships between structures and processes using linguistic articulations, rather than mathematical or computational formulisms. In disciplines like neuroscience and psychology, verbal and experimental models are often tested using statistical models. These models can then drive the formulation of more refined verbal, experimental, mathematical, or computational models. This nesting of and interaction between models is central to theoretical development. As philosopher of biology, Richard Levin, writes:
A satisfactory theory is usually a cluster of models. These models are related to each other in several ways: as coordinate alternative models for the same set of phenomena, they jointly produce robust theorems; as complementary models they can cope with different aspects of the same problem and give complementary as well as overlapping results; as hierarchically arranged “nested” models, each provides an interpretation of the sufficient parameters of the next higher level where they are taken as given.
Relying on a cluster of models is necessary because, in the words of Levin again:
…all models are both true and false. Almost any plausible proposed relation among aspects of nature is likely to be true in the sense that it occurs (although rarely and slightly). Yet all models leave out a lot and are in that sense false, incomplete, inadequate. The validation of a model is not that it is “true” but that it generates good testable hypotheses relevant to important problems.
Most research in biopsychology and neuroscience is conducted using verbal, experimental, and statistical models.[4] This means that these disciplines benefit from the conceptual and methodological strengths of these types of models, but also that they suffer from the inherent partialities of these model types. They also stand to benefit less from the strengths of other types of models. Verbal models, for example, generally lack precision with regards to both the exact mechanisms and their parameterization that could produce the dynamics and outcomes specified by the model. Verbal models also tend to either contain hidden assumptions or be imprecise in their definitions and specifications of the concepts that make up the model. Indeed, the imprecision of verbal models motivates much work in philosophy, which tries to highlight and remedy this difficulty in different domains. Experimental and statistical models can test mechanistic hypotheses, but conceptual and practical constraints limit these to one or a very small number at a time. It is exceedingly difficult to use such mechanistically limited models to develop a sufficiently rich explanation of complex systems like the brain. This is well articulated in the article, The tale of the neuroscientists and the computer: why mechanistic theory matters. The article author advocates that “…every neuroscientist should at least possess literacy in modeling as no less important than, for example, anatomy”.
Verbal models are, however, very portable. They can be relatively easily communicated either out loud (talking to each other) or in writing (such as in this text). Verbal representations are also excellent tools for compressing information. For example, you can refer to a rich illustration of an object in three words: “It’s a pipe”. Like all data compression strategies, whether it’s in a digital music file or the “mean” as a metric in statistics, compression entails loss of information. But it also affords access. We can talk with each other much more easily than we can share computational and mathematical models. We’re all raised to communicate in language. Ideas can spread through our social and neural networks with breath-taking speed, helping to scaffold deeper and broader understandings of the world.
Critically, all of our understanding is mediated by models and expressed through representations. This is not just true of scientific understanding, but also our own moment-to-moment understanding of our world. We saw how our perceptual world is mediated by predictive models in Chapter 3. We opened this chapter with how we express our understanding through a verbal representation, “It’s a pipe”, of an imagistic representation (the painting). To say that all of our understanding is mediated by models and expressed through representations is to echo anthropologist and semiotician Gregory Bateson, who wrote:
We say the map is different from the territory. But what is the territory? Operationally, somebody went out with a retina or a measuring stick and made representations which were then put on paper. What is on the paper map is a representation of what was in the retinal representation of the man who made the map; and as you push the question back, what you find is an infinite regress, an infinite series of maps. The territory never gets in at all. …Always, the process of representation will filter it out so that the mental world is only maps of maps of maps, ad infinitum.
Not only are we networks all the way down, but our mental worlds are maps all the way down. The partiality of individual representations, models, and perspectives compels us to search for more. I enthusiastically encourage you to continue exploring a range of diverse perspectives—within and beyond yourself, and within and beyond a discipline—in your pursuit of understanding. Enjoy!
- Representations—such as images and words—approximate only select dimensions of the object or system of representation. This is why Magritte’s painting of a pipe can accurately be captioned, “This is not a pipe”.
- The differences between a representation and the object or system of representation is also called the map-territory distinction. Importantly, the map is not the territory. However, it can be easy to either conflate or misunderstand the relationship between the two.
- Neuroimaging figures from fMRI and PET are often misunderstood as representing brain activity when a person performs a task. This misunderstandinng may contribute to neuromyths about how the brain works.
- Neuroimages are derived using the subtraction technique and therefore represent the difference between a control task and an experimental task. Control tasks are rarely described in media reports. This makes it very difficult to critically evaluate a claim about the purported function of a brain area.
- Neuroimages are representations of blood flow, an indirect measure of neural activity. As a result, while fMRI and PET have high spatial resolution, they also have low temporal resolution.
- Different neuroscience methods engender different tradeoffs, as do all representations.
- Models are representations which help describe, explain, and predict the functioning of a system.
- All models are wrong, but some are useful.
- Each chapter of this book presented models. There were several different types of models, such as computational, mathematical, and verbal models.
- Research in cognitive, behavioral, and systems neuroscience generally relies on verbal, experimental, and statistical models. Literacy in other types of models would improve theories in neuroscience.
- All of our understanding—not just scientific understanding but everyday understanding—is mediated by models and expressed through representations.
- By seeking out and exploring new models, in whichever discipline or arena of life they can be found, we improve our understanding of ourselves and of our world.
Media Attributions
- 21336510168_533aa7e7bd_o © outtacontext is licensed under a CC BY-NC-ND (Attribution NonCommercial NoDerivatives) license
- FMRI scan during working memory tasks © John Graner, Neuroimaging Department, National Intrepid Center of Excellence, Walter Reed National Military Medical Center, 8901 Wisconsin Avenue, Bethesda, MD 20889, USA, Public domain, via Wikimedia Commons is licensed under a Public Domain license
- PET Image © Jens Maus (http://jens-maus.de/) is licensed under a Public Domain license
- 1-s2.0-S0959438817302465-gr2_lrg © Thomas Deffieux, Charlie Demene, Mathieu Pernot, Mickael Tanter is licensed under a CC BY (Attribution) license
- Torczyner, H. (1977). Magritte ideas and images (p. 71). H.N. Abrams. ↵
- The warmer colors in this image correspond to grey matter of the brain, containing cell bodies of neurons and glial cells, while the cooler colors correspond to areas in which there is either white matter or ventricles. ↵
- There are actually a number of different experimental and statistical methods for isolating functional brain activity, but what they all have in common is the making or modeling of contrasts between different states. ↵
- This is certainly true of cognitive, behavioral, and systems neuroscience, if not also cellular and molecular neuroscience. ↵